Difficult challenges and choices face today’s healthcare industry. Researchers, clinicians and administrators have to make important decisions—often without sufficient data. Graal Systems offers its Data Platforms to make healthcare data available and actionable.
Researchers explore the genetic architecture of cancer cells. Nurses and physicians monitor intensive care patients. Administrators submit reimbursement claims before patients leave the hospital. Graal Systems is transforming big data analytics in healthcare and medicine.
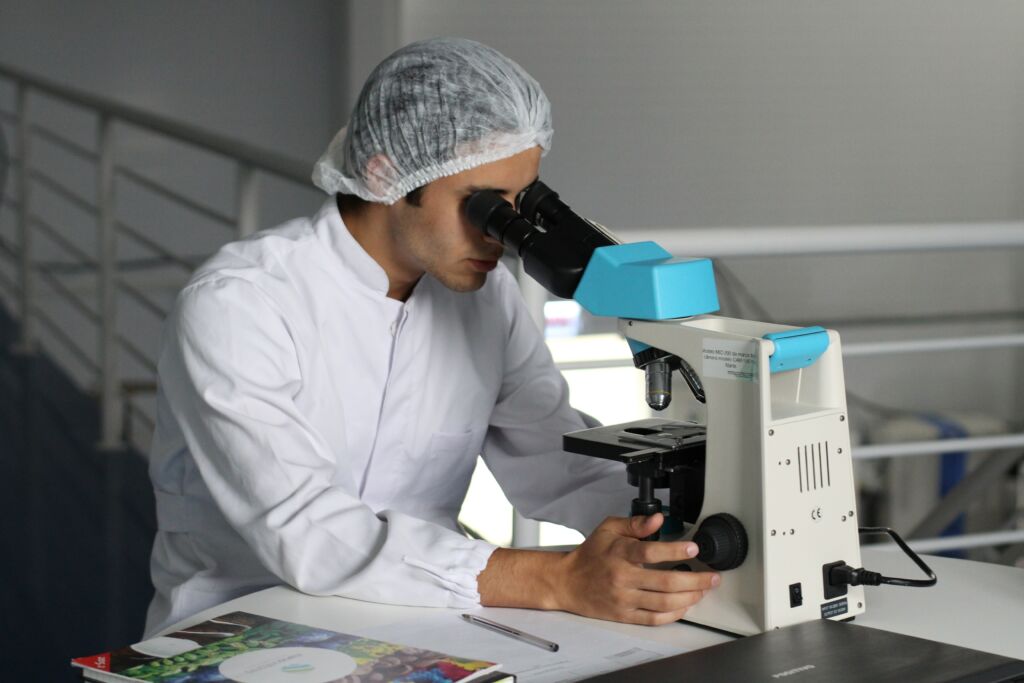
Use Cases
If we read that a given drug is “40% effective in treating cancer,” another interpretation could be that the drug is 100% effective for patients with a certain genetic profile. However, genomic data is Big Data. The data in a single human genome includes approximately 20,000 genes. Stored in traditional data platforms, this is the equivalent of several hundred gigabytes. Combining each genome with one million variable DNA locations produces the equivalent of about 20 billion rows of data per person.
Researchers at major universities and teaching hospitals are performing big data analytics in genomics with Graal Data Platform as the cost-effective, reliable platform for storing genomic data and combining that with other data on demographics, trial outcomes, and real-time patient responses. They are adopting Graal DataFlow to stream that data into Graal Platform for real-time decisions and long-term cohort analyses. Connected Data Platforms help those doctors learn which drugs and treatments work best for groups of patients across the genetic spectrum.
In a typical hospital setting, nurses do rounds and manually monitor patient vital signs. They may visit each bed every few hours to measure and record vital signs but the patient’s condition may decline between the time of scheduled visits. This means that caregivers often respond to problems reactively, in situations where arriving earlier may have made a huge difference in the patient’s wellbeing.
New wireless sensors can capture and transmit patient vitals far more frequently than human beings can visit the bedside, and these measurements can stream into our platform. Caregivers can use these signals for real-time alerts to respond more promptly to unexpected changes. Graal uses this data accumulated over time for healthcare predictive analytics, feeding algorithms that proactively help predict the likelihood of an emergency even before it could be detected with a bedside visit.
Patients with heart disease can be closely monitored while they are in a hospital, but when those patients go home, they may skip their medications or ignore dietary and self-care instructions given by their doctor when they left the hospital.
Congestive heart failure causes fluid retention, which leads to weight gain. In one innovative program at UC Irvine Health, patients could return home with a wireless scale and weigh themselves at regular intervals. Algorithms running in Graal’ healthcare predictive analytics determined unsafe weight gain thresholds and alerted a physician to see the patient proactively, before an emergency re-admittance was necessary.
Autism spectrum disorders affect 1 in 100 children at an annual cost estimated at more than $100 billion. The condition can be detected through behavior at eighteen months, but more than 1 in 4 cases are still undiagnosed at 8 years of age. A small number of clinical testing facilities are oversubscribed, with long wait lists. The most common diagnostic test typically takes 2.5 hours to administer and score.
Dr. Dennis Wall is Director of the Computational Biology Initiative at the Harvard Medical School. In this presentation, he describes a process his team developed for low-cost, mobile screening for autism. It takes less than five minutes and relies on the ability to store large volumes of semi-structured data from brief in-home tests administered and submitted by parents. Wall’s lab also used Facebook to capture user-reported information on autism.
Artificial intelligence running on those huge data sets helps maximize efficiency of diagnosis without loss of accuracy. This approach, in combination with data storage on our platform, can be used for other innovative machine learning diagnostic processes.
Medical and scientific researchers at universities live by the “publish or perish” code. Data supporting a given paper used to be appended in an Excel spreadsheet, but many of today’s data sets are just too large. Nevertheless, supporting data sets must be perpetually available in association with its paper. If the data disappears, the paper becomes unsubstantiated.
Universities can use a cluster running Graal Data Platform as a cost-effective, perpetual storage platform for its scientists’ data. Big data in medicine’s easy and open querying capabilities allow scientific colleagues to share data, validate it and reuse it for more downstream research.
Hospitals have begun to use radio-frequency identification (RFID) to track equipment and medicines that move throughout their facilities. RFID scans of an item or device can capture their contents, location, manufacture date, order numbers, and shipping data. One innovative hospital group was able to determine how long its doctors stood in front of sinks to wash their hands (reducing the likelihood of disease transmission).
In the short run, this data can help utilize medicines before their dates of expiration or quickly locate an important piece of equipment. Over time, historical data on how medicines, equipment and doctors interact provides valuable information for healthcare predictive analytics and helps in planning purchases, training staff and improving operational efficiency.